Event Description
Abstract:
People shift their visual attention to gather and prioritize information from their surroundings, helping them navigate complex environments. Understanding these attentional shifts involves decoding the features that guide where attention is directed (spatial areas of focus) and when attention shifts (timing). Decoding these processes can aid applications from interface design to medical diagnosis. However, prior models have not fully explored the underlying factors addressing these aspects. In this dissertation, we study the factors that guide visual attention across diverse image types, spanning natural images, graphic design documents, and whole slide images (WSIs) of cancer tissues, while also predicting visual attention based on these factors.
First, we propose a method to quantify object recognition uncertainty as a factor influencing spatio-temporal attention (where and when) in natural images. We found that it plays a larger role than bottom-up saliency in guiding visual attention. Second, we analyze graphic design documents such as webpages, comics, posters, mobile UIs, etc., which differ from natural images in that they are designed to convey specific messages or elicit desired viewer response. We propose a unified and interpretable deep learning model that predicts both static and dynamic visual attention behavior (addressing where and when) by integrating document layout and content saliency as factors, enhancing attention prediction performance. Finally, in the domain of digital pathology, we investigate pathologists' attention during their examination of giga-pixel WSIs of prostate cancer with an objective to aid in the development of computer-assisted pathology training and clinical decision support systems. Using a digital microscope interface, we collected the largest known dataset of pathologist attention, which allows us to study the factors that guide their spatial and temporal attention patterns (where and when) and develop predictive models. Our study explores key factors guiding their attention, including magnification, slide staining, the nature of the diagnostic task, and their expertise. Motivated by this analysis, we propose deep learning models to solve two tasks: 1) predicting pathologist attention via spatial (heatmaps) and spatio-temporal (scanpaths) models, and 2) inferring pathologist expertise level, both essential technical components towards developing an AI-assisted pathology training pipeline.
Speaker:
Souradeep Chakraborty
Location: New Computer Science Bldg., Room 220
Zoom Link: https://stonybrook.zoom.us/j/
Meeting ID: 975 528 8447
Passcode: 338037
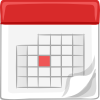