Event Description
Abstract: Sea ice is crucial to Earth's climate, Arctic communities, and ecosystems, yet climate change is driving significant losses, threatening polar stability. Quantifying the long-term impacts of a declining sea ice cover requires tools which improve climate-timescale prediction and bring new understanding of climate interactions. In this talk, I discuss how meeting this challenge requires a multi-disciplinary approach. Climate models, while essential, suffer from systematic biases due to missing or inaccurate physics, leading to uncertainty in future projections. I show how data assimilation (DA) offers a statistical framework for integrating satellite observations with climate models to quantify systematic sea ice model errors. Using convolutional neural networks (CNNs), we can learn these errors based on the model's atmospheric, oceanic, and sea ice conditions--what I term a state-dependent representati
IACS Seminar Speaker: William Gregory, Princeton University
Location: IACS Seminar Room
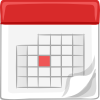