Event Description
Abstract:
Photorealistic editing of human facial expressions and head articulations remains a long-standing topic in the computer graphics and computer vision community. Methods enabling such control have great potential in AR/VR applications where a 3D immersive experience is valuable, especially when this control extends to novel views of the scene in which the human subject appears. Traditionally, 3D Morphable Face Models (3DMMs) have been used to control the facial expressions and head pose of a human head. However, the PCA-based shape and expression spaces of 3DMMs lack the expressivity. They cannot model essential elements of the human head such as hair, skin details, and accessories such as glasses that are paramount for realistic reanimation. In this thesis, we present a set of methods that enables facial reanimation, starting from editing expressions in still face images to creating fully controllable neural 3D portraits with control over facial expressions, head pose, and viewing direction of the scene using only casually captured monocular videos from a smartphone to finally achieving studio-like quality from the said monocular captures.
First, we propose a method for editing facial expressions in near-frontal facial images through the unsupervised disentangling of expression-induced deformations and texture changes. Next, we extend facial expression editing to human subjects in 3D scenes. We represent the scene and the subject in it using a semantically guided neural field. This enables control over the subject's facial expressions and the viewing direction of the scene they're in. We then present a method that learns, in an unsupervised manner, to deform static 3D neural fields using facial expression and head-pose dependent deformations, enabling control over facial expressions and head pose of the subject along with the viewing direction of the 3D scene they're in. Next, we propose a method that makes the learning of the aforementioned deformation field robust to strong illumination effects, which adversely impact the registration of the deformation. We then propose an extension of this unsupervised deformation model to 3D Gaussian splatting by constraining it using a 3D morphable model, resulting in a rendering speed of 18 FPS--a 100x speed improvement over prior work. Finally, we propose a method that bridges the quality gap between 3D portraits created using in-the-wild monocular data and multi-view studio capture data. We accomplish this using a two-stage method. First, we train a StyleGAN to relight and inpaint in-the-wild face texture maps (with strong illumination effects and incompletely captured regions). Next, we both reconstruct and generate identity-specific facial details that may be poorly captured in the in-the-wild captures. Once trained, we can generate studio-like complete avatars from monocular phone captures.
Speaker: Shahrukh Athar
Zoom Link:
https://stonybrook.zoom.us/j/94228500743?pwd=RqOBgG6tbJkKaFBlWFwBkYFX0VRovV.1
Meeting ID: 94228500743
Passcode: 661599
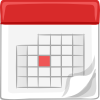