Event Description
Abstract: The rapid growth of observational data presents unprecedented opportunities to enhance both the predictability and mechanistic understanding of Earth systems. However, fully harnessing big Earth data needs computational frameworks that bridge the gap between physics-based models and machine learning. In this talk, I will first demonstrate how AI methods can significantly improve the prediction of environmental systems. Despite their predictive accuracy, machine learning models often lack physical interpretability, limiting their ability for scientific inquiry. To address this, I will introduce the developed hybrid, differentiable modeling framework that unifies physical models with machine learning in an end-to-end trainable system. This framework autonomously learns from large observations while maintaining physical clarity. The machine learning components can be seamlessly embedded into physical backbones to assimilate multi-source data, support automatic parameterization, and represent uncertain processes. I will showcase applications of this framework in simulating and understanding the terrestrial water cycle and its interactions with ecosystems at continental and global scales. This talk will highlight how differentiable modeling not only improves the modeling ability in both data-rich and data-scarce scenarios, but also provides a systematic pathway to enhancing model structures, deciphering uncertain physical relations, and facilitating knowledge discovery in Earth system sciences.
IACS Seminar Speaker: Dapeng Feng, Stanford Univeristy
Location: IACS Seminar Room
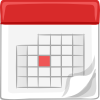