Location
Event Description
Virtual Talk: Metadata Matters: Robust Document Classification via Adaptation Methods for Text-driven Public Health by Xiaolei Huang
Zoom link to follow.
Abstract: Document classifiers have been widely applied in solving health-related issues, such as suicide prevention, flu vaccination surveillance and disease diagnosis. However, document metadata including time, gender, age and location has an enormous impact on robustness of
document classifiers. Language varies across the metadata bringing both challenges and opportunities to build reliable document classifiers. For example, online written language changes over time, and males and females express opinions differently. This talk describes how to use domain adaptation to integrate temporal and user demographic factors into document classifiers. By adapting knowledge of how language varies across the metadata, models can learn generalized representations of language through the metadata-invariant embeddings.
This approach will lead to metadata-adapted document classifiers and can also extend to personalize classification models by user embedding.
Bio: Xiaolei Huang is a 4th-year PhD candidate in Information Science at the University of Colorado, Boulder. He is currently a visiting scholar at the Johns Hopkins University. His research interests are in Natural Language Processing, Machine Learning and Public Health. Particularly, he focuses on domain adaptation, cross-lingual transfer learning, user modeling and fairness.
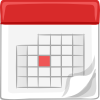