Event Description
Abstract: Millions of individuals living in disadvantaged communities are burdened by poverty, illegal drug activities, health concerns, and the lack of reliable and affordable access to facilities (e.g., schools, hospitals, and transit stations). To address these societal problems efficiently with broad support, initiatives have called to engage agents (e.g., residents, community leaders, or stakeholders) and consider their preferences on community improvement decisions to make collective community decisions. In this talk, we will focus on our ongoing AI-empowered collective decision-making approaches to improve the accessibility of individuals to facilities by (a) locating facilities to provide essential services and (b) strengthening existing infrastructures via structural modifications (e.g., constructing new roads, bridges, multi-use paths, or shuttle services) subject to individuals' preferences on the locations of the facilities and which communities to improve access, respectively. In particular, we will discuss our (theoretical and algorithmic) studies on modeling these approaches under several settings (e.g., accounting for fairness and agent preferences) and designing fair, transparent, strategy proof, and (approximately) optimal mechanisms to elicit (true) individual preferences and determine collective community decisions in order to improve facility accessibility. Finally, we will discuss other ongoing and future collective decision-making efforts in urban planning and public health (i.e., our recent studies on substance use research) to improve communities.
Bio: Hau Chan is an assistant professor in the School of Computing at the University of Nebraska-Lincoln. He received his Ph.D. in Computer Science from Stony Brook University in 2015 and completed three years of Postdoctoral Fellowships, including at the Laboratory for Innovation Science at Harvard University in 2018. His main research lies in multi-agent aspects of AI for Society and Social Good, focusing on developing modeling and algorithmic foundations for tackling societal problems involving agents and predicting agent behavior in societal contexts, leveraging AI, game theory, mechanism design, and machine learning to better inform policymaking and (collective) decision-making. His team has been addressing societal challenges and fairness issues in various domains, including security (e.g., reducing vulnerability), public health (e.g., reducing substance use and homelessness), and urban planning (e.g., improving accessibility to public facilities), collaborating with domain experts. His research has been supported by NSF, NIH, and USCYBERCOM. He has received several Best Paper Awards at SDM and AAMAS and distinguished/outstanding SPC/PC member recognitions at IJCAI and WSDM. He has given tutorials and talks on computational game theory and mechanism design at venues such as AAMAS and IJCAI, including an Early Career Spotlight at IJCAI 2022. He has served as co-chairs for the AI and Social Good Track, Demonstration Track, Student Activities, Doctoral Consortium, Job Fair, Scholarships, Finance, and Diversity & Inclusion Activities at AAAI, AAMAS, and IJCAI.
Location: Old Computer Science, room 1310
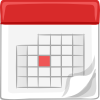