Researchers at Stony Brook University and Salesforce AI Research reveal why professional writers often feel disappointed with Large Language Models, proposing a manually polished model that can aid in aligning a Machine’s Language to our own.
Stony Brook, NY, Apr 16, 2025 — The rise of large language models (LLMs) has heralded a new era in artificial intelligence writing assistants, revolutionizing how we write, communicate, and express ideas. From assisting in scientific research and crafting persuasive arguments to sparking creativity in literary works, LLMs have shown great promise. Yet, despite their potential, key differences between LLM-generated and human-written text persist, raising questions about their effectiveness in producing high-quality, human-like writing.
Recent research has illuminated these challenges, revealing that while LLMs such as GPT, Claude, and Llama excel at generating text, they often fall short in certain creative and nuanced writing domains. A comprehensive study led by Assistant Professor Tuhin Chakrabarty, Department of Computer Science at Stony Brook, and aided by professional writers, provides insight into these shortcomings, offering a roadmap for improving the alignment between machine-generated content and human writing. The paper was nominated for Best Paper and Honorable Mention Awards at CHI 2025.
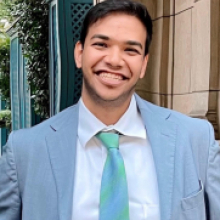
Tuhin Chakrabarty
Tuhin said, “One significant issue we noticed is that LLM-generated text often suffers from a lack of originality and variety.” The widespread use of LLMs in writing tasks contributes to what is referred to as "algorithmic monoculture," where content becomes overly homogenized. This is why writing with LLMs tends to result in repetitive, clichéd expressions and a lack of rhetorical depth. The texts often fail to demonstrate the complex, layered narrative techniques that characterize human creativity, frequently resorting to “telling” instead of “showing.”
To address these concerns, the researchers set out to better understand the specific idiosyncrasies of LLM-generated writing. They recruited 18 professional writers to edit LLM-generated text using a comprehensive taxonomy of edit categories, developed based on expert writing practices. This taxonomy categorizes undesirable traits found in LLM-generated content, such as clichés, redundant phrases, and poor structural choices.
Focusing on literary fiction and creative non-fiction, this team of professional writers aimed to tackle genres that challenge LLMs with their emotional nuance and sophisticated language use. By concentrating on paragraph-level edits, the researchers were able to strike a balance between granularity and scope, reducing annotator fatigue and allowing for more cohesive improvements.
From this research, the team compiled the LAMP (Language Model Authored, Manually Polished) corpus, which contains 1,057 LLM-generated paragraphs, edited by professional writers according to the proposed taxonomy. These edited texts — totaling 8,035 fine-grained edits — offer valuable insights into the kinds of adjustments needed to improve machine-generated writing.
An analysis of the LAMP corpus showed that the three LLMs studied (GPT-4, Claude 3.5 Sonnet, and Llama 3.1 70b) did not outperform each other in terms of writing quality. In fact, the team found consistent weaknesses across all models, including the overuse of certain structures, lack of stylistic diversity, and failure to convey subtle narrative nuances.
Building on previous research, the study explored methods for improving the alignment between LLM- and human-written text. One promising approach is using "edit-based alignment," where LLM-generated responses are paired with edited versions, highlighting areas for improvement. By teaching LLMs to detect and rewrite their own idiosyncrasies, these edits can reduce undesirable traits and better align with human writing standards.
“Our research provides a roadmap for improving LLM-generated writing by identifying consistent weaknesses and offering practical solutions for better alignment with human writing preferences,” Tuhin said. “It underscores the importance of human-AI collaboration, where experts guide the overall structure and flow of a piece, while AI handles lower-level writing details.”
As LLM-based writing tools become increasingly integrated into our daily lives, it is crucial to ensure that they enhance human creativity rather than diminish it. While these models have made significant strides, they still face challenges in producing text that reflects the richness and diversity of human expression.
With continued research and development, LLM-based writing assistants may one day be able to seamlessly collaborate with human writers, improving both productivity and the overall quality of written content.