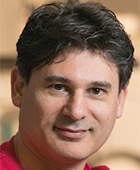
Life Sciences Building
Neurobiology & Behavior
Office: Room 513
Phone
Interests
Biography
Giancarlo La Camera studied Theoretical Physics at the University of Rome "La Sapienza" and received a Laurea (M. Sci.) in 1999. He went on to obtain a PhD in Neurobiology from the University of Bern in 2003. Between 2004 and 2008 he was a visiting fellow at the National Institute of Mental Health, where he performed research on the neural basis of cognitive functions. He then returned to the University of Bern where he focused on the topic of reinforcement learning in populations of spiking neurons. In early 2011 he joined the faculty of Stony Brook University as an Assistant Professor of Neurobiology & Behavior and was promoted to the rank of Associate Professor with tenure in 2017.
Research
The laboratory is interested in the neural basis of sensory and cognitive processes. These include memory, decision making, and more recently the chemosensory processes involving taste perception. The laboratory performs theoretical research comprising mathematical and computational approaches. Priority is given to finding biologically plausible models, often in terms of populations of spiking neurons. Recently, with this approach we have characterized the metastable nature of ongoing and evoked activity in several cortical areas (most notably the primary gustatory cortex), and worked out some of its consequences for neural coding. We hope that uncovering the common basis of ongoing and evoked activity can tell us much about how cortical networks are organized and function. The investigation of what type of sensory and cognitive functions can be subserved by metastable (rather than stable) neural activity is also a central effort of the lab.
The lab is also interested in the theory of learning, and therefore in modeling synaptic plasticity and coming up with learning rules for neural circuits. Recently, we have obtained results on the compound problem of segmenting a sensory stream and making decisions based on the segmented input. This was achieved by reinforcement learning in a network of spiking neurons, and is a first step towards understanding how an agent learns to identify the 'features' of its environment that are relevant for making decisions.
In addition to seeking a theoretical understanding of these issues, we team up with other research groups in the Department of Neurobiology and elsewhere to test our models against empirical data.