Event Description
George Em Karniadakis received his SM and PhD from Massachusetts Institute of Technology. He was appointed lecturer in the Department of Mechanical Engineering at MIT in 1987 and subsequently he joined the Center for Turbulence Research at Stanford/Nasa Ames. He joined Princeton University as assistant professor in the Department of Mechanical and Aerospace Engineering and as associate faculty in the program of applied and computational mathematics. He was a visiting professor at Caltech in 1993 in the Aeronautics Department and joined Brown University as associate professor of applied mathematics in the Center for Fluid Mechanics in 1994. After becoming a full professor in 1996, he continues to be a visiting professor and senior lecturer of Ocean/Mechanical Engineering at MIT. He is an AAAS fellow (2018), fellow of the Society for Industrial and Applied Mathematics (2010), fellow of the American Physical Society (2004), fellow of the American Society of Mechanical Engineers (2003) and associate fellow of the American Institute of Aeronautics and Astronautics (2006). He received the Alexander von Humboldt award in 2017, the Ralf E Kleinman award (2015), the J. Tinsley Oden Medal (2013), and the CFD award (2007) from the US Association in Computational Mechanics. His h-index is 103, and he has been cited over 52,000 times.
Abstract:
Karniadakis will present a new approach to develop a data-driven, learning-based framework for predicting outcomes of physical and biological systems, governed by PDEs, and for discovering hidden physics from noisy data. He will introduce a deep learning approach based on neural networks (NNs) and generative adversarial networks (GANs). He will also introduce new NNs that learn functionals and nonlinear operators from functions and corresponding responses for system identification. Unlike other approaches that rely on big data, here we learn from small data by exploiting the information provided by the physical conservation laws, which are used to obtain informative priors or regularize the neural networks. He will demonstrate the power of PINNs for several inverse problems in fluid mechanics, solid mechanics and biomedicine including wake flows, shock tube problems, material characterization, brain aneurysms, etc., where traditional methods fail due to lack of boundary and initial conditions or material properties. He will also present a new NN, DeepM&Mnet, which uses DeepOnets as building blocks for multiphysics problems, and he will demonstrate its unique capability in a 7-field hypersonics application.
To register and for more information, click here.
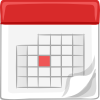