Location
Event Description
Johannes Hachmann, University of Buffalo Assistant Professor of Chemical Engineering presents Making Machine Learning Work in Chemistry
The use of modern machine learning, informatics and data mining approaches is a relatively new development in the chemical and materials domain. These techniques have been exceedingly successful in other application fields, and since there is no fundamental reason why they should not have a similarly transformative impact on chemical and materials research, there is now a concerted effort by the community to introduce data science in this new context. However, adapting techniques from other application domains for the study of chemical and materials systems requires a substantial rethinking and redevelopment of the existing methods.
In this presentation, we will discuss our work on designing advanced, physics-infused neural network architectures, the fusion of unsupervised clustering with supervised regression for local ensemble models, active and transfer learning techniques, bootstrapping approaches to minimize our training data footprint, methods to increase the applicability domain of data-derived models and automated hyperparameter optimization.
Biosketch: Johannes Hachmann is an Assistant Professor of Chemical Engineering at the University at Buffalo (UB), the Director of the Engineering Science in Data Science graduate program, a Core Member of the UB Computational and Data-Enabled Science and Engineering graduate program, and a Faculty Member of the New York State Center of Excellence in Materials Informatics. He earned a Dipl.-Chem. degree (2004) after undergraduate studies at the universities of Jena and Cambridge, M.Sc. (2007) and Ph.D. (2010) degrees in Chemistry from Cornell University, and he conducted postdoctoral research at Harvard University before joining the UB faculty in 2014. The research of the Hachmann Group fuses (first-principles) molecular and materials modeling with virtual high-throughput screening and modern data science (i.e., the use of database technology, machine learning and informatics) to advance a data-driven discovery and rational design paradigm in the chemical and materials disciplines. One of the centerpieces of the group's efforts is the creation of an open, general-purpose software ecosystem for the data-driven design of chemical systems and the exploration of chemical space. This work was recognized with a 2018 NSF CAREER Award.
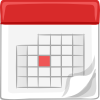