Location
Event Description
A talk by Jerome Zhengrong Liang entitled, Machine Learning from Original Images to Texture Patterns: A Paradigm Shift from Non-Medical Application to Medical Diagnosis.
Abstract: Artificial intelligence (AI) research for medical diagnosis started soon after human began to use computer, initially called artificial neural network (ANN) and now convolutional neural network (CNN). ANN has been mainly explored to classify the experts' handcrafted features from the original (or raw) images, while CNN has been mainly explored directly on the raw images for both tasks of extracting abstract features and classifying the features.
Experimental evidences have been shown that CNN can be trained by a large number of the raw images with experts' scores (or labels) to match or even surpass the experts' performance for both non-medical and medical diagnosis applications. However, the performances of the CNN models as well as the experts on medical diagnosis dropped dramatically when the labels of the raw images were replaced by the corresponding medical pathological reports.
Accumulated medical knowledge reveals that the lesion heterogeneity is a footprint of lesion evolution and ecology, and the heterogeneity is an indicator of lesion progress and response to medical intervention. The heterogeneity can be reflected by the image contrast distribution (or texture patterns) across the lesion volume. Image textures have been shown as an effective descriptor of the lesion heterogeneity for computer-aided diagnosis.
Can we map the raw images into texture patterns (or images) and train CNN to learn from the texture images? This question is the central theme of this presentation with application to CT Colonography or virtual colonoscopy, a game from AlphaGo to PolypGo.
Bio: Jerome Zhengrong Liang, PhD, IEEE Fellow
Imaging Research and Informatics Laboratory
Department of Radiology, Stony Brook University
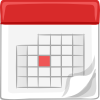