Location
Event Description
Speaker Petar Djuric
Refreshments will be provided
Deep Gaussian processes: Theory and applications
Petar M. Djurić
Department of Electrical and Computer Engineering
Stony Brook University
Abstract: Gaussian processes are an infinite-dimensional generalization of multivariate normal distributions. They provide a principled approach to learning with kernel machines and they have found wide applications in many fields. More recently, with the advance of deep learning, the concept of deep Gaussian processes has emerged. Deep Gaussian processes can be viewed as multilayer hierarchical organizations of Gaussian processes that are equivalent to infinitely wide multiple layer neural networks. Deep Gaussian processes have improved capacity for prediction and classification over standard Gaussian processes, while models based on them continue to allow for full Bayesian treatment and for applications when the amount of available data is limited. The theory of recent progress in deep Gaussian processes will be presented and some applications will be provided.
Biosketch: Petar M. Djurić received the B.S. and M.S. degrees in electrical engineering from the University of Belgrade, Belgrade, Yugoslavia, respectively, and the Ph.D. degree in electrical engineering from the University of Rhode Island, Kingston, RI, USA. He is a SUNY Distinguished Professor and currently, he is a Chair of the Department of Electrical and Computer Engineering, Stony Brook University, Stony Brook, NY, USA. Djurić was a recipient of the IEEE Signal Processing Magazine Best Paper Award in 2007 and the EURASIP Technical Achievement Award in 2012. From 2008 to 2009, he was a Distinguished Lecturer of the IEEE Signal Processing Society. He was the Editor-in-Chief of the IEEE Transactions on Signal and Information Processing over Networks (2015-2018). Djurić is a Fellow of IEEE and EURASIP
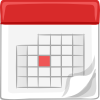