Event Description
Title: AI-Driven Target Selection Methods for Touch and Gaze Input
Abstract: Accurately selecting targets is an essential aspect of Human-Computer Interaction. Erroneous selections can cause tedious undo and redo actions. Additionally, some selection errors are non-reversible and can lead to undesirable consequences. However, high-accuracy target selection remains a challenge on touchscreen devices due to the small target size and imprecise touch inputs, and in gaze interaction because of the gaze tracking noise and no easy-to-use selection action. We first propose ReLM, a Reinforcement Learning-based Method for touchscreen target selection. ReLM can automatically show suggestions and require a second touch if the input is ambiguous, and can directly select a target candidate when the input is certain. Our empirical evaluation shows that ReLM reduces the error rate from 6.92% to 1.63%, and the selection time from 2.23s to 1.59s over Shift, an existing suggestion-based method. Compared to BayesianCommand, a direct selection-based method, our ReLM reduces the error rate from 3.64% to 0.89%, while increasing the selection time by only 200 ms. Secondly, we investigate how to improve target selection performance for gaze interaction. We propose BayesGaze, an eye-gaze based target selection method. It accumulates the signal of each gaze point for selecting a target calculated by Bayes Theorem, and uses a threshold mechanism to determine the target selection. Our investigation shows that BayesGaze improves target selection accuracy and speed over a dwell-based selection method, and the Center of Gravity Mapping method.
All are welcome. Here is the zoom meeting link:
https://stonybrook.zoom.us/j/
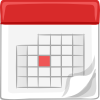