From using the New York Times Connections word game to decoding the basics of language and cognition, AI researchers from Stony Brook University present their out-of-the-box approaches at this year's EMNLP conference on how we can improve machines’ understanding of human language.
Artificial Intelligence (AI) tools like ChatGPT and Google Gemini are transforming how content is created, and creating new problems around privacy and truth. At the same time, these tools can be incredibly useful in compiling information and drafting messages.
A group of Stony Brook students this spring took a close look at potential benefits and risks of using AI tools, particularly as they could enhance or hinder advocacy efforts for social causes. The students created a guide for advocacy groups that will help those groups consider how, when or if to use AI in their work.
Department of Ecology and Evolution Professor Ross Nehm has been selected to serve on the National Science Foundation funded committee “Advancing AI in Science Education: A Comprehensive Approach to Equity, Inclusion, and Three-Dimensional Learning.”
Stony Brook, NY, Oct 21, 2024 - SBU Professor Ross Nehm, a leading researcher on the use of AI in student assessment, is one of 15 international scholars selected to serve on this NSF-funded committee, which is charged with establishing norms, frameworks, and guidelines for AI-involved science education research.
Harsh Trivedi, a doctoral researcher at Stony Brook University, was recently invited to present his work at the White House. His project, AppWorld, promises to revolutionize how we automate daily tasks in our digital lives.
Stony Brook, NY, Oct 19, 2024 - Stony Brook University Professor Niranjan Balasubramanian and Ph.D. researcher Harsh Trivedi were invited to represent the university at the White House Office of Science and Technology Policy (OSTP) launch event to celebrate the first allocation of the NAIRR Pilot.
The Provost’s Spotlight Talks feature eminent visitors to the university as well as Stony Brook faculty members who have recently been recognized for outstanding contributions in their field.
spotlightimage
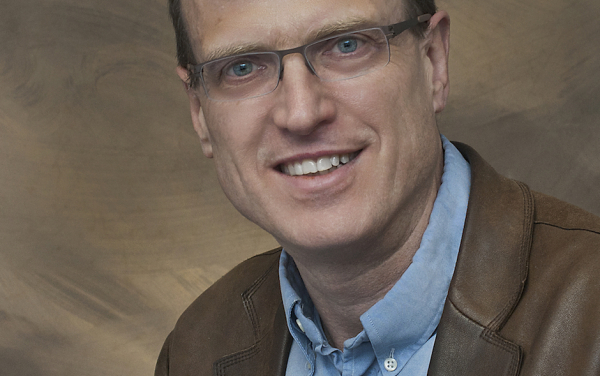
Spotlight Link
Weight
Research project supported by NIH uses AI to predict how much trust individuals and communities have in U.S. counties.
Stony Brook, NY, Oct 12, 2024 - In the United States, public confidence in institutions has been declining over the past several decades. It is easy to imagine how these low levels of trust might lead to negative social, political, and economic outcomes, especially in the heat of the 2024 presidential elections. This is why measuring trust consistently and at scale is crucial.
Stony Brook University professor Robert J. Harrison, founding endowed director of the Institute for Advanced Computational Science (IACS), has been appointed interim executive director of New York State’s first-in-the-nation Empire AI Consortium.
Collaborative research to test method in opioid use disorder.