spotlightimage
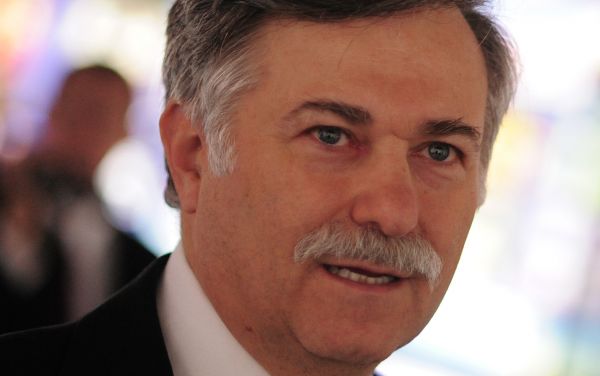
Spotlight Link
Weight
Stony Brook, NY, August 15, 2024 - Stony Brook University’s Computer Science Department is pleased to announce the hiring of two exceptional faculty members: Tuhin Chakrabarty and Paola Cascante-Bonilla.
Stony Brook University researchers develop GlanceWriter, a hands-free typing tool for people traveling without a keyboard and individuals with motor disabilities.
Have you ever noticed how your iPad camera follows you when you’re FaceTiming a friend? Now imagine that camera studying your eye movements to help you type words as you look at a keyboard.
Virtual 360-degree tours have become the norm in real estate, more so since the pandemic made it impossible for buyers to visit homes in person.